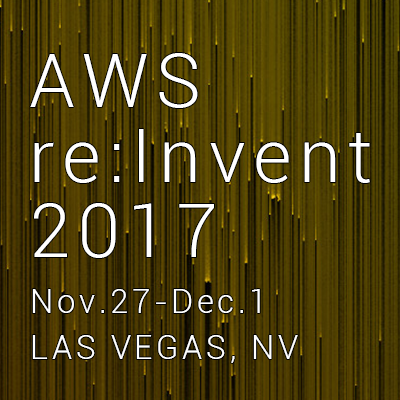
【レポート】ALX315 – Test Automation for Alexa Skills #reinvent
はじめに
re:Invent 2017のセッション「ALX315 - Test Automation for Alexa Skills」についてご紹介します。
セッション概要
Automated testing is an essential part of assuring the quality and functional state of your software. One of the biggest challenges in automating your testing can be the simulating user interaction with your UI. For Alexa custom skills, users generally interact via multi-step conversations, which result in a request-response flow to your endpoint. The right set of tools and APIs can help you tackle the complexity of simulating such interactions in an automated fashion. This session demonstrates how to create conversational scripts that can be executed against an Alexa skill endpoint to validate the skill’s output against predefined assertions. You'll leave this talk armed with practical knowledge of how to establish powerful test automation suites for your Alexa skills, helping you ensure that your new skills behave exactly as you expect, and that you don’t accidentally break things while working on new versions. Bring your own laptop and AWS account.
セッション資料
Agenda
- Basics
- Test execution
- Test validation
- Test automation
- Wrap up
Basics
Why should you test your Alexa skill?
なぜテストが必要なのか
What makes testing Alexa skills different?
Alexa skills のテストは何が違うのか。
- ASR(自動音声認識)
- NLU(自然言語理解)
- Skill Lambda
- Request handlers
- 音声は非線形入力 → 100%のテストガバレッジは諦める → それらは70億の音声がある
- 常に学習しているクラウド常にビルドし、行動を変え続ける → どのようにシミュレーションするか
- あなたのskillは非決定性なユーザーインタラクションを対処する → テストがもっとも一般的な状況をカバーするにはどうするか
- ユーザーはskillとやりとりするのに障害はない → 予期せぬユーザー入力を使用してスキルの堅牢性をテストする必要がある。
What tools can I use to test my Alexa skill?
Alexa silllをテストするのにどのツールを使用するか。
- マニュアルテスト用のAlexa対応デバイス
- デバイスなしでテストする EchoSim.io
- Service simulator in the developer console
- ASK CLI to access testing APIs via SMAPI
- Custom extensions and OS tools
Test execution
ワークショップとしてLabが用意されていますので実際に手を動かして確認できます。
alexa/skill-sample-nodejs-test-automation
Skill Management API (SMAPI) and ASK CLI
Alexaスキルおよび関連リソースをプログラムで管理やテストがAPI操作できる。 ASK CLIはコマンドラインアプリケーションで、ユーザーがSMAPIを呼び出して公開するためのAlexaスキルのcreate、update、test、submitを可能にします。
Lab1 は、「SMAPIとASK CLIを使用してAlexaカスタムスキルをセットアップ」がゴールです。
Github repoをクローンしたディレクトリに移動して、ask deploy
とコマンドを実行して終わりです。
ask api list-skills
と実行するとスキルが作成されていることが確認できます。
デプロイ
$ ask deploy -------------------- Create Skill Project -------------------- Profile for the deployment: [default] Skill Id: amzn1.ask.skill.cefc668-****-****-****-************ Skill deployment finished. Model deployment finished. [Info]: No lambda functions need to be deployed.
スキルの確認
$ ask api list-skills { "skills": [ { "lastUpdated": "2017-12-04T09:47:42.925Z", "nameByLocale": { "en-US": "Number guessing game" }, "skillId": "amzn1.ask.skill.cefc668-****-****-****-************", "stage": "development" }, [...] ] }
ASK CLIを使用してSkillを作成することができました。
Service simulator
developer portalはデバイスなしでテストする為のツールを提供します。 voice simulatorでは、フレーズをプレーンテキストまたはSSMLで入力し、Alexaがテキストをどのように話すか聞くことができます。 Service simulatorを使用すると、発話を入力してから、サービスに送信されたJSON要求とサービスが返すJSON応答を確認できます。 レスポンスを再生して、Alexaの言葉を聞くこともできます。
Lab2 は、「Service simulatorを使用してAlexaスキルをテストする」がゴールです。
発話を入力してくださいに「the number is ten」の文言を入力して「Number gussing game」を呼び出します。
レスポンスを確認するとoutputSpeechにAlexaがユーザーに返す言葉が含まれています。
{ "version": "1.0", "response": { "outputSpeech": { "ssml": "<speak> Sorry. That’s not correct. My number is lower than <say-as interpret-as=\"cardinal\">10</say-as> <p>I am thinking of a number between one and <say-as interpret-as=\"cardinal\">10</say-as>. What is your guess?</p> </speak>", "type": "SSML" }, "speechletResponse": { "outputSpeech": { "ssml": "<speak> Sorry. That’s not correct. My number is lower than <say-as interpret-as=\"cardinal\">10</say-as> <p>I am thinking of a number between one and <say-as interpret-as=\"cardinal\">10</say-as>. What is your guess?</p> </speak>" }, "shouldEndSession": false } }, "sessionAttributes": { "hasAlreadyBeenHere": 1, "number": 1 } }
「the number is one」で正解しました。
{ "version": "1.0", "response": { "outputSpeech": { "ssml": "<speak> Great. That’s it. You want more? </speak>", "type": "SSML" }, "speechletResponse": { "outputSpeech": { "ssml": "<speak> Great. That’s it. You want more? </speak>" }, "shouldEndSession": false } }, "sessionAttributes": { "hasAlreadyBeenHere": 1 } }
Echosim.ioで実際に発話でのテストも可能です。
open number guessing
と言ってスタートします。
Skill Invocation API
Skill Invocation APIは特定のスキルのAWS LambdaまたはサードパーティのHTTPSエンドポイントを呼び出します。 応答が成功すると、エンドポイントとの間で送受信されるペイロードと同様に、エンドポイントが呼び出されたかに関する情報も含まれます。このAPIを使用して、開発者のスキルを呼び出すことができます。 呼び出されるスキルは、アカウントで有効にする必要があり、開発段階にある必要があります。 スキルエンドポイントへのコールは10秒後にタイムアウトします。
Skill Simulation API
Skill Simulation APIは、スキルの実行をシミュレートする非同期APIです。 成功したレスポンスには、シミュレーションリソースの場所を含むヘッダーが含まれます。 このAPIを使用して、開発者のスキルをシミュレートすることができます。 シミュレートされるスキルは、アカウントで有効にする必要があり、開発段階にある必要があります。 このAPIは、ユーザーあたりの同時リクエストをサポートしていません。
Lab3はSkill Invocation API and Skill Simulation APIを使用してAlexaスキルをテストします。
Invoke a skill with ask-cli
Lab2と同様にAmazon developer consoleで"start number guessing"と入力してASKボタンを押します。Lambdaリクエスト
のtextboxの内容をコピーして空の./assets/launch.jsonファイルに貼り付けて変更を保存します。
次に、コマンドラインで、ask-cliを使用してInvocation APIを使用して、スキルで同じ要求を発生させます。 このリポジトリのルートフォルダ内から次のコマンドを実行します。
$ ask api invoke-skill -e NA -s amzn1.ask.skill.cefc668-****-****-****-************ -f assets/launch.json { "status": "SUCCESSFUL", "result": { "skillExecutionInfo": { "invocationRequest": { [...] "sessionAttributes": { "hasAlreadyBeenHere": 1, "number": 6 } } }, "metrics": { "skillExecutionTimeInMilliseconds": 110 } } } }
Alexaが現在のスキルセッションで思う数字らしきものがあるので、Service Simulatorでthe number is six
としたところ、Great. That’s it. You want more?
と応答がありました。
Simulate user interaction
音声でskillに発話するのと同じようにコマンドラインで実施してみます。
$ ask api simulate-skill -l en-US -s amzn1.ask.skill.cefc668-****-****-****-************ -t 'start number guessing' { "id": "7c502961-****-****-****-************", "status": "IN_PROGRESS" }
Simulation APIは非同期に処理するためスキルからの実際の応答を取得するために使用できるIDを使います。 idをコピーして
$ ask api get-simulation -s amzn1.ask.skill.cefc668-****-****-****-************ -i 7c502961-****-****-****-************ { "status": "SUCCESSFUL", "result": { "skillExecutionInfo": { "invocationRequest": { [...] "sessionAttributes": { "hasAlreadyBeenHere": 1, "number": 2 } } }, "metrics": { "skillExecutionTimeInMilliseconds": 111 } } } }
レスポンスされたペイロードを確認するとInvocation APIとほぼ同じ内容が返ってきたことがわかります。
Test validation
Test client
典型的なスキルの会話は、複数のステップから構成されています。 それはスキル呼び出しの振り付けです。 Alexaクラウドサービスは、スキルから返されるセッション属性を追跡し、次のスキル要求に渡します。 このようなセッション処理とサービスコールオーケストレーションを模倣するインスタンスが必要です。
Lab4はテストSDKをセットアップし、テストクライアントをインスタンス化します。
Lab4ではCloudFormationを使用してセットアップしました。
Assertions
Assertionsはテスト実行の実際の結果と比較されるテスト実行(skill invocationなど)の期待結果を定義するためにソフトウェアテストで使用されます。 Assertionsが期待を満たさない場合、テストケースは失敗します。 テストクライアントSDKには、output-speechやreturned cardなど、スキルレスポンスアセットに関する多くの事前定義されたAssertionsが付属しています。
Lab5ではskillを使用してシングルターンの会話を設定し、期待される反応についていくつかのアサーションを立てます。
InvocationApi
実行例)ログ
->[FALSE] response.shouldEndSession == false is TRUE. ->[TRUE] response.shouldEndSession == false is TRUE.
SimulationApi
実行例)ログ
Asynchronous processing in progress. Keep on polling for result of transaction with id 98e0f5f8-fbeb-4d01-858f-b4355a997f64 ->[TRUE] response.shouldEndSession == false is TRUE.
SimulationApiの場合は、idがが使用されていることがわかりました。
Multiturn conversations
スキルセッションは、通常、ユーザーとの複数の対話から構成されます。 1つのセッション内のユーザーのすべてのやり取りを会話と呼びます。 会話をシミュレートするには、テストクライアントが個々のステップを個別に定義し、順次フロー(テストパス)で配置する必要があります。 アサーションは、ターンとスキルレスポンスごとに個別に行うことができます。
Lab6ではスキルを使用して複数回会話を設定し、Test SDKツールを使用して期待される応答をいくつかアサーションします。
Multipath conversations
ユーザーが自分のスキルを習得する方法はたくさんあり、次のいずれかに基づいて異なります。スキルは、特定の状況(月曜日が日付、ユーザーが初心者、パーソナライズされたコンテンツまたはランダム化されたコンテンツなど) )または多くの決定のうちの1つを行うユーザーは行いません。 テストクライアントは、これらの要因を考慮して、非確定的なテストパスを実行できます。
Lab7ではスキルを使用して複数回会話を設定し、Test SDKツールを使用して期待される応答をいくつかアサーションします。 それに加えて、特定の条件が満たされたときに条件付きパスを入力します。
Run conversation scripts periodically
Lab8ではスキルの定期的なテストの実行をスケジュールし、テストが失敗した場合にアラームを設定します。
What are the benefits?
- Conversation scriptsはスキルが提供するすべての機能をカバーする技術仕様
- ライフサイクルに沿ってスキルを実施する
- これらのスクリプトは、Alexaスキルの期待された機能と実際の機能を確実に遵守し、いつでも「設計どおりに動作する」ようにします。
- Conversation scriptsはVUI-diagramsから派生したもの
- 適切な会話パスのカバレッジが与えられれば、スクリプトは基礎となるVUIデザインに準拠してスキルの実装を管理できます。
- テストドリブン開発の道を切り開いている。
- 高品質なソフトウェアを構築するベストプラクティスです。
おわりに
Alexaの自動テストについて学べました。ユーザーのやりとりをシミュレートすることでAlexaの品質を保証することができるのかと思います。ワークアウトもあり大変充実したセッションかと思いました。
ではまた。